It Is All About Data: A Survey on the Effects of Data on Adversarial Robustness
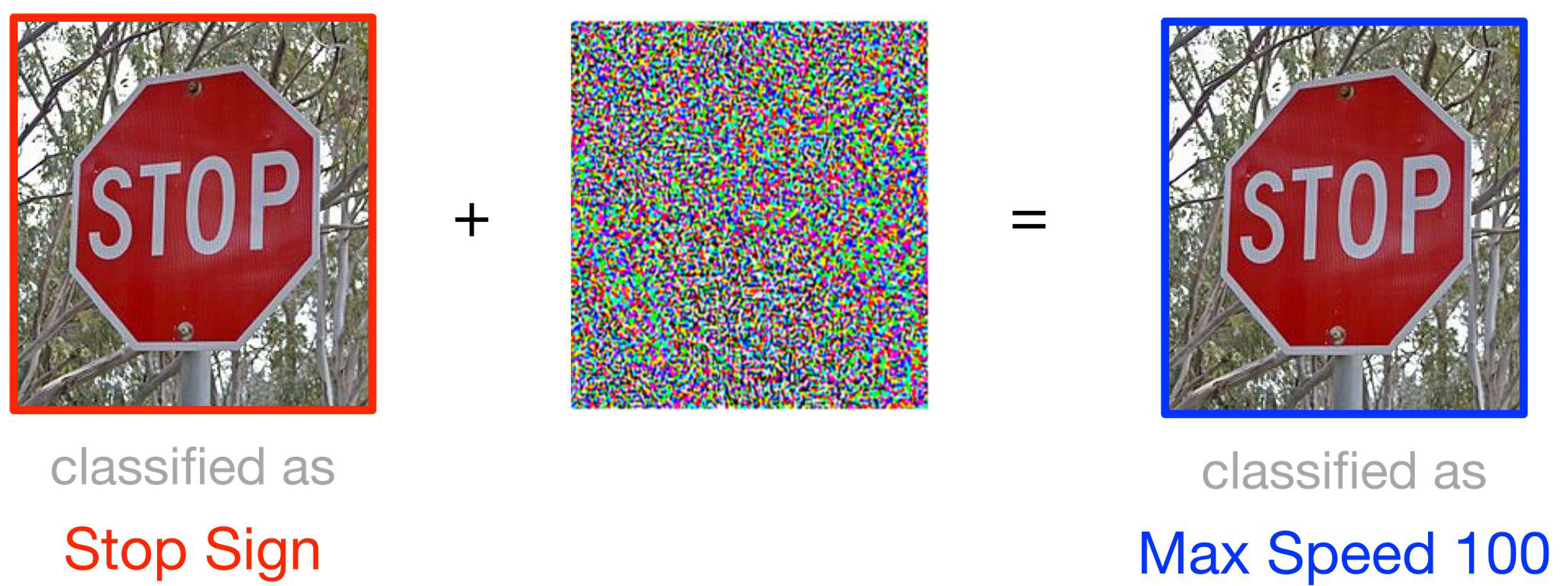
Adversarial examples are inputs to machine learning models that an attacker has intentionally designed to confuse the model into making a mistake. Such examples pose a serious threat to the applicability of machine-learning-based systems, especially in life- and safety-critical domains. To address this problem, the area of adversarial robustness investigates mechanisms behind adversarial attacks and defenses against these attacks. This survey reviews a particular subset of this literature that focuses on investigating properties of training data in the context of model robustness under evasion attacks. It first summarizes the main properties of data leading to adversarial vulnerability. It then discusses guidelines and techniques for improving adversarial robustness by enhancing the data representation and learning procedures, as well as techniques for estimating robustness guarantees given particular data. Finally, it discusses gaps of knowledge and promising future research directions in this area.
Publication
Peiyu Xiong, Michael Tegegn, Jaskeerat Singh Sarin, Shubrahneel Pal and Julia Rubin. It Is All About Data: A Survey on the Effects of Data on Adversarial Robustness. Special Issue on Trustworthy AI in ACM Computing Surveys (CSUR), 2023. [pdf, bibtex]